Enhancing Accuracy of Position and Distance Measurements for Connected Vehicles based on Modified Ap
Accurate positioning of vehicles is a critical element of autonomous and connected vehicle systems. Most of other studies heavily focused on enhancing simultaneous localization and mapping (SLAM) methods, i.e., computationally constructing or updating a map of an unknown environment and tracking an object within the map. This paper provides a method that can, in addition to existing SLAM or relevant methods, enhance the raw measurements of position and distance and therefore. The basic idea of this study is to identify and update error distribution of multi-source raw data measurements by combining all available information. A modified Approximate Bayesian Computation method was incorporated. The estimation is conducted real-time based, and the learning process will try to keep improving the accuracy of estimation. The results show that the proposed model noticeably improves the accuracy of position and distance measurements. The estimated error distribution can also be used for improving results of other post-processing techniques which require assumptions of certain type of error distributions. A similar approach can also be utilized to enhance accuracy of other sensors or measurements in connected vehicle or relevant systems, where multi-data sources are available.
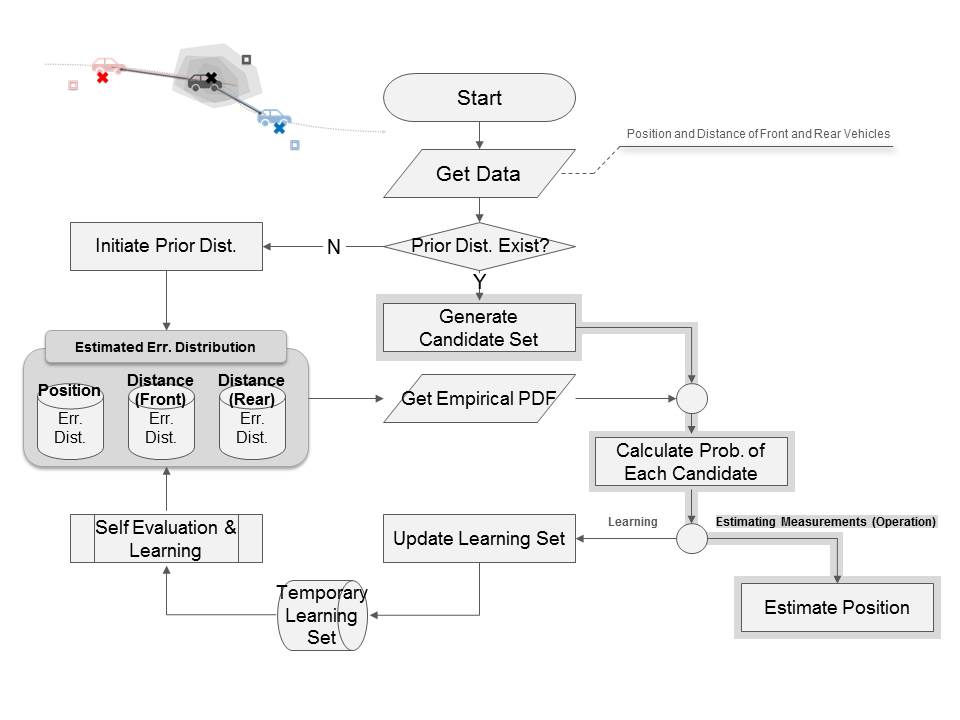